In the world of speech AI, the professional and personal use cases are limitless and result in greater efficiency, collaboration, and productivity. However, a major concern arises when companies and people consider the data security and data privacy of an AI model once it’s in application. How can automatic speech recognition (ASR) tools protect one’s most sensitive information if it’s capturing and storing all that a person may say?
While many speech AI solutions have faced this challenge, aiOla has approached it with novelty and innovation – delivering a first-of-its-kind model with built-in named entity recognition (NER) capabilities. If this all sounds like gibberish or you’re simply intrigued, read on to learn more as we will uncover this approach to ethical AI practices and how it resolves many key challenges for businesses.
What is Named Entity Recognition?
Before we get into the details, let’s consider first how automatic speech recognition (ASR) works. ASR applies machine learning and artificial intelligence to transform spoken words into transcribed and readable text.
Natural language processing (NLP) enables computers to understand human language through statistical modeling, deep learning and machine learning.
While all of these technologies work together in an AI model to make speech AI possible, there’s still something missing- and that’s how to anonymize and/or protect personal information from being stored or used just because it was spoken aloud as part of the initial audio recording.
To exemplify the risk here: imagine a healthcare worker transcribing a patient’s sensitive information, including their name, date of birth, diagnosis, and billing information. Most companies have to go back to the transcribed text to remove these specifics after it has been said and transcribed.
In the meantime, the stored data is at risk of a breach or hack. In fact, this delicate situation happened when 9 million patients had their data stolen after a US medical transcription firm was attacked.
To address these concerns, we have to consider the use of named entity recognition (NER). Named entity recognition is an aspect of natural language processing that can identify predetermined categories in a body of text.
For example, this can include:
- Individuals’ names
- Expressions of times
- Medical codes
- Monetary percentages
- Locations
- Organizations, etc.
NER can classify different words in text. Here’s a few ways in which it works:
- POS Tagging: POS tagging, or parts-of-speech tagging, assigns labels to identify parts of speech, i.e. nouns, verbs, and adjectives.
- Word Embeddings: This captures semantic meanings by translating words or phrases into numerical vectors of fixed size, so it’s easier to process for machine learning models.
- Corpus: Corpus refers to the collection of texts used to train NER models for linguistic analysis. It can include journals, social media posts, news articles, etc.
Ultimately, NER turns unstructured data into structured data so it is easier for analysis and the creation of organized datasets. With this result, companies gain access to better information retrieval, automated data entry, and content recommendations, to name a few advantages.
Yet, with all the upsides, the main challenge still exists, namely: how does a company protect private data from being transcribed in the first place? Since NER takes place as part of the audio processing, the data still exists and may be at risk of being exposed.
What is Whisper-NER and How is it Different Than What’s Out There?
aiOla developed a solution to protect private and sensitive data during the transcription stage. The Whisper-NER model recognizes and masks sensitive information. To put it into action, all users have to do is list what names of entities they wish for the model to identify, such as “Patient Name” or “Patient Address,” for example, along with the audio file.
The model then transcribes the audio while masking the entities at the same time, so no personal data and information ever gets stored, even temporarily. In turn, companies can rest assured that they are enhancing security, privacy, and compliance, all without any extra effort or work.
Since businesses deal with many different types of audio and applications for speech AI, it may be the case where this level of privacy isn’t necessary. The Whisper-NER model is flexible and can be configured to identify and tag entities without masking them, as well. This is especially helpful for use cases like inventory management, inspections, quality control, and the like, where all details are relevant to the task at hand.
The Importance of Speech Compliance
For any person or business that has utilized speech AI or is interested in doing so, the concerns surrounding speech compliance comes into mind inevitably.
Data is a double edged sword– it gives businesses what they need to make swift and smart decisions, but it also introduces risk if used incorrectly, stolen, or stored improperly. With the rise of data and the application of artificial intelligence (AI), speech compliance is being molded by government agencies and business leaders alike.
Businesses and technology providers are responsible for speech compliance and speech analytics compliance. Companies can train their staff on how to properly manage data. Similarly, they look to their speech recognition tools to possess top-notch security, including encryption and data storage protection.
Now, with aiOla’s Whisper-NER model, there’s one more less concern to worry about- named entities are inherently screened and protected from the get go of transcription.
As aiOla’s VP of Research Gill Hetz explains:
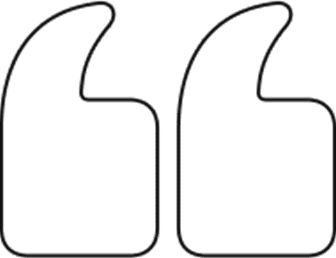
“Whisper-NER is the first open-source AI model that not only detects and masks sensitive data but can ensure that sensitive information is never generated in the first place.” He adds, “Whisper-NER operates as a zero-shot solution, combining both tasks in one elegant step. This innovation not only boosts performance but also strengthens ethical AI practices, fostering trust in the secure and responsible collection of speech data.”
How it Works and Where to Find It
Built on top of OpenAI’s Whisper, Whisper-NER was trained using a synthetic dataset that combines large amounts of synthetic speech with open NER text datasets. By doing so, the model was able to learn both transcription and entity recognition simultaneously.
Want to try it for yourself?
aiOla is releasing Whisper-NER as an open-source model on GitHub and Hugging Face, making this advanced solution accessible to the community, with a demo available here for users to explore.
Closing Thoughts
With the embedded named entity recognition that comes with aiOla’s Whisper-NER models, companies no longer have to question if their customers’ and business’ private and sensitive data is ever at risk. Compliance and security are inherent, so businesses can continue to use speech AI to get more done, with one less worry.
FAQs